The C-Suite Lacks Confidence in HR Data Analytics. But Why?
Majority of executives say blame for faulty analytics lies with IT
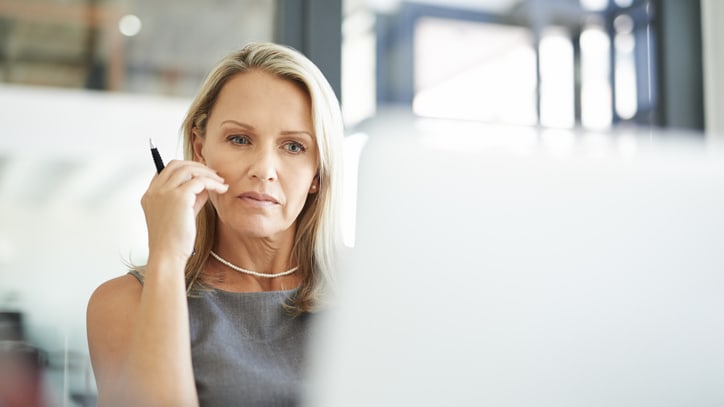
Capital investment in HR data analytics is at an all-time high. But while organizations recognize the technology's power to help organizations make more-informed decisions, business leaders are also concerned about the trustworthiness of these tools.
Recent research from Dutch professional-services firm KPMG indicates a significant trust gap. In the survey of 2,190 senior executives from Australia, Brazil, China, France, Germany, India, South Africa, the U.K. and the U.S., just 35 percent said they have a high level of trust in their organization's use of data and analytics. Another 40 percent said they had reservations about relying on the data and analytics they produce, and 25 percent admitted they have either limited trust or active distrust in their data and analytics. Nearly all respondents (92 percent) worry about the impact flawed data could have on their company's business and reputation.
"Executives and managers are being asked to make major decisions based on the output of an algorithm that they didn't create and don't always fully understand," said Thomas Erwin, global head of KPMG International's Lighthouse, the firm's center of excellence for data, analytics and intelligent automation. "As a decision-maker, you really need to have confidence that the insights you are getting are reliable and accurate, but many of these executives can't even be sure if their models are of sufficient quality to be trusted. It's an uncomfortable situation for any decision-maker to be in."
[SHRM members-only online discussion platform: SHRM Connect]
Most companies have incomplete data, said Kevin Wheeler, founder and president of the Future of Talent Institute, a San Francisco-area think tank. "Analytics functions are new to most companies and they're trying to gain credibility, so they're analyzing anything and everything and presenting tons of data in a shoddy way. I'm not surprised a CEO looks at that and says, 'What is this?' and 'Why are you telling me this?' "
Even generally accepted metrics like the gross domestic product and national productivity measurements have very low validity, Wheeler added.
"When it comes to HR data, the important criteria include how was it gathered, how large is the dataset and how long has it been analyzed? In many cases we have to wait to find out whether or not the data are useful. We're very early in understanding which analytics are important and which aren't."
Another issue is placing blind trust in quantitative analytical data—which Wheeler calls "thin data." The traditional source of hire metrics is a good example.
"No one really knows how to measure for source of hire," he said. "It's either self-reported by the candidate or reported by a tool. But let's say I saw the job ad on Facebook and then went to a party and decided to apply. I returned home and applied through the employer's careers site. The source of hire will be logged as the careers site, but that's not true. That chain becomes really hard to measure."
Quality of hire is another one, he said. "There are many definitions for it—none are wrong, but neither are any universally correct. The definitions for these metrics are poor and not generally agreed upon."
Who Is Responsible?
Over half of respondents (55 percent) to the KPMG study said that the company's technology function and service providers are currently held chiefly responsible for poor business decisions based on insight from faulty data analytics. Just 33 percent said responsibility would fall on the core business, such as the CEO or CHRO or functional departments. Sixty-two percent said IT should hold responsibility for poor analytics-led business decisions.
"The leaders' impulse is to absolve the core business for decisions made with machines," Erwin said. "This impulse is understandable given technology's legacy as a support service, the difficulty of assessing the trustworthiness of analytics, and the tendency to give all things technical to the 'experts.' "
But he believes this is a mistake. "As organizations begin to think about behavior of machines as parallel to the behavior of people, they should also consider new models of governance to support the leaps in trust that the human/machine workforce requires," Erwin said. "At a fundamental level, accountability of machines must be held firmly by the CEO and functional leaders."
Wheeler believes it's a shared responsibility. "Data analysts need to be candid and upfront about how good they believe the data are, how big the sample was, how reliable it was and how they collected it. They need to be honest about that."
Another issue arises when the requesters of analytics aren't sure what to ask for, he said. "If you don't know what you want to know, you'll get faulty data. And what you want to know must be realistic. Can it even be shown by analytics?"
Wheeler suggested that data analysts push back if they think the request is not fully baked, and take the time to test reliability before presenting analytics.
The Human Element
Ultimately, HR needs to be able to appreciate the value of "thick data"—thin data merged with the experience, knowledge and intuition that only humans can bring, Wheeler explained.
"The best that numbers alone can do is give us insight," he said. "If you just look at it from a shallow, quantitative perspective and take action based on that, it's probably a mistake. When you intelligently consider data to augment your own decision-making, the data become very valuable. I'm a strong advocate for using hiring assessments to provide some concrete data about a candidate, but I'm not going to base my hiring decision on it. I'm still going to use my gut."
Was this article useful? SHRM offers thousands of tools, templates and other exclusive member benefits, including compliance updates, sample policies, HR expert advice, education discounts, a growing online member community and much more. Join/Renew Now and let SHRM help you work smarter.
An organization run by AI is not a futuristic concept. Such technology is already a part of many workplaces and will continue to shape the labor market and HR. Here's how employers and employees can successfully manage generative AI and other AI-powered systems.