Data Analytics Make Understanding Quality of Hire Possible
Outcome data dispels popular notions about core recruiting practices
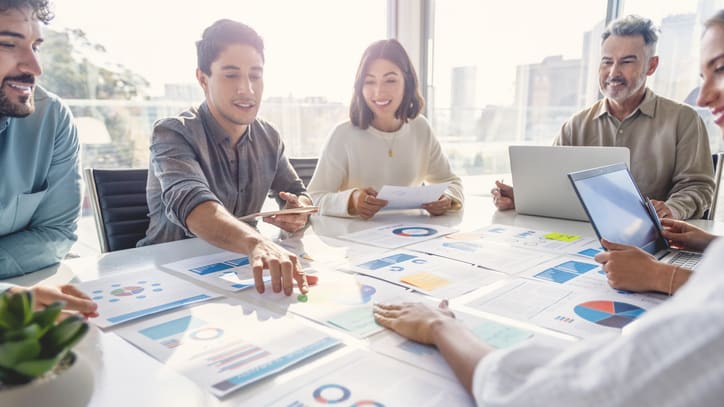
Quality of hire is generally considered to be the most meaningful recruiting metric—and the most difficult to calculate. Because of the ever-increasing power of data analytics, however, hiring managers and recruiters are gaining a more accurate snapshot of the key components that connect data from the hiring process with post-hire business outcomes. And some of those connections may be surprising, according to an analysis of workforce data conducted by Crosschq, a recruiting software and data analytics firm in San Francisco.
Known for years as the Holy Grail of talent acquisition, quality of hire is the value that a new employee contributes to the success of the company. It's the deepest-impact metric HR can utilize to increase workforce productivity, improve retention and realize organizational goals. But it's been frustratingly hard to get a fix on.
"Quality of hire has historically been elusive," said Mike Fitzsimmons, CEO of Crosschq. "It has typically been based on someone working from an Excel spreadsheet, making a subjective decision, looking at performance and tenure. Every company had its own interpretation, and there was no real process or machine precision behind it."
Putting a numeric value on the intangibles that directly relate to quality of hire is difficult, according to John Feldmann, senior communications specialist at Insperity, an HR outsourcing firm in Kingwood, Texas. "The problem usually stems from an insufficient measuring system, or no system at all," he said.
"It's primarily a data problem," Fitzsimmons agreed. "Most companies have fragmented and siloed data sources, from the ATS [applicant tracking system] and other prehire platforms to the HRIS [HR information system] and performance management system."
But another problem is more human, he added. "Who has the time to determine quality of hire? Who owns it, and who is accountable for making it better? For decades, the core KPIs [key performance indicators] for talent acquisition have been butts in seats and time-to-fill."
Unlike time-to-fill or cost-per-hire, determining quality of hire with unbiased accuracy has proved to be very difficult, if not impossible, without data analytics technology.
With data analytics, "understanding the quality of a hiring decision requires an automated, real-time and credible feedback loop between recruiting and employee outcomes," said Kerry Wang, CEO and co-founder of Searchlight, a San Francisco-based talent intelligence company. "In the past, it's been arrived at through a painful, expensive process of trial and error, and some never get it right. People teams are usually siloed from recruiting teams, it's difficult to pick the right factors for quality, and there's little bandwidth to administer the data manually, let alone generate the insights."
Vendors like Crosschq and Searchlight aggregate and process large amounts of data and use machine learning to make sense of it. "By consolidating data from HRIS and ATS systems, payroll and benefits platforms, assessment and interview tools, and joining it with outcome data from performance management systems, culture and engagement platforms, sales management tools, and others, we build and analyze employee profiles that span the entirety of their life cycle—from a person's first interaction with a company as a candidate all the way through their exit interview," Fitzsimmons said. The profiles are enhanced with survey data, collected from co-workers and managers.
Technology can also push the objective from simple measurement to proactive recommendations based on the insights, Wang said. "The real value for an organization is in the predictions and interventions that can drive better selection, retention and performance, and the predictive analytics that operationalize quality of hire."
Feldmann said it's important to first identify the primary elements that constitute a good hire for the company, and then work backward. "For example, what helps the company achieve long-term goals? Once these elements are identified, the process can be reverse-engineered in order to determine what qualities a candidate must possess that will yield these outcomes."
Some long-standing assumptions about the hiring process can also be dispelled by quality-of-hire data, according to a recently released report based on the 24 million hiring decisions processed by Crosschq. The report's insights about internal referrals, interviewing practices and prehire assessments may surprise some.
Referrals Come Up Short
It's commonly believed that internal referrals are the most fruitful source of quality candidates. Although this can be true at some organizations, the Crosschq data showed that quality of hire for internal referrals was 26 percent below the mean.
"There are a number of contributing factors," Fitzsimmons said. "Some hiring teams typically demonstrate less scrutiny in the selection process when evaluating an internal referral than they do when evaluating an outside applicant. In addition to referral bias, there are also other considerations around economic incentives for the referrer that can have an impact."
The data showed that when organizations remove financial incentives like referral bonuses, quality of hire goes up dramatically, while the quantity of referrals drops.
Candidates from job boards also scored below the mean in quality of hire, while recruiter-sourced employees typically had above-average quality-of-hire scores.
"Overall, employee referrals should be weighted very low when measuring hire quality, as there are more analytical and numbers-based indicators that can determine the metric more accurately," Feldmann said.
Most Interviewers Lack Training
The Crosschq data showed only a 9 percent correlation rate of interview scores to quality of hire, revealing that interviewers' perceptions of winning candidates are not aligned with organizational goals.
"Interviewing is weighted heavily in hiring decisions, but it may not be the best way to predict post-hire performance or fit," Fitzsimmons said. "The research revealed that most interviewers are not skilled or experienced at the task. When looking at the few interviewers who did 12 interviews per year or more, there was a greater correlation between higher interview scores and higher post-hire performance evaluations."
While interview scores may not predict post-hire success, they can help identify patterns, Feldmann said. "Some candidates may excel in job interviews but fail to deliver on expectations once hired. However, if this happens regularly, changes must be made. Employers that are seeking to boost their quality of hire must go back to square one, which starts with the interview process. By identifying specific knowledge, skill sets or personality traits that current or former employees possess that lead to success in the role they're looking to fill, they can then tailor interview questions to better determine post-hire success."
Many Hiring Assessments Not Predictive
Similar to interviewing, the data showed that many of the popular assessments used in the hiring process are not good predictors of new-hire quality. Some even had an inverse correlation to quality of hire. "It is paramount to assess the assessments," Fitzsimmons said. "Not only are many candidate assessments not predictive, but they also can negatively affect candidate experience and also increase time-to-hire. Don't waste time and money on pricey assessments that add noise to an already complex hiring program."
Certain prehire assessments have proved to be more predictive of success, Fitzsimmons added. "Role-specific assessments such as technical assessments for engineers or mock sales pitches for account executives have shown high correlations between those scores and quality of hire."
An organization run by AI is not a futuristic concept. Such technology is already a part of many workplaces and will continue to shape the labor market and HR. Here's how employers and employees can successfully manage generative AI and other AI-powered systems.